AI stock predictions have reshaped how you spot investment opportunities, promising faster insights than ever before.
Today, hedge funds, retail traders, and even corporate CFOs rely on artificial intelligence to help forecast price movements, identify undervalued companies, and fine-tune their timing on trades.
This article will walk you through how AI systems predict prices, where they thrive, and where they fall short.

How AI Systems Predict Prices
AI stock prediction usually relies on machine learning models trained on years, sometimes decades, of historical data. These systems ingest everything from daily price movements to quarterly earnings reports, global news sentiment, analyst upgrades, and even chatter on X (formerly known as Twitter).
The goal? To identify patterns that humans might miss and use those to forecast where a stock could head next.
For example, natural language processing (NLP) models scan financial results and earnings call transcripts to detect sentiment.
Deep learning models then weigh signals alongside charts, moving averages, RSI levels, and dozens of other indicators to generate a probability-based prediction — for instance, maybe a 74% chance a stock goes up 10% in the next 30 days.
Some platforms also use reinforcement learning, where the algorithm learns from real trades over time, adjusting its strategy based on what worked and what didn’t. That’s a big leap forward from traditional backtesting, since AI is constantly evolving.
AI Stock Prediction in Action
Take C3.ai’s latest financial results: In Q2 of 2025, the enterprise AI software provider reported a 29% year‑over‑year revenue surge to $94 million.
That revenue acceleration came after C3.ai switched from flat subscriptions to a consumption‑based revenue model, billing customers by computing usage rather than a fixed fee.
In one sense, their success story illustrates the power of AI applications — tools that can forecast server demand and adjust pricing on the fly. Yet the company’s $66 million net loss that same quarter shows the limits of algorithmic forecasting: even the best models struggle when costs or policy shifts outpace predictions.
Behind those headline figures lies a story of stock growth tied closely to new enterprise agreements. C3.ai closed 191 deals in fiscal 2024, up 52% from the prior year, fueling investor optimism and boosting the company’s market cap. Still, understanding how AI tools inform those deals — and where they fall short — matters more than ever if you want to make informed choices about where to allocate your capital.
Revenue Acceleration and Stock Growth
When companies embrace AI to drive demand forecasting, they often see rapid revenue acceleration. By analyzing pilot project success rates, C3.ai’s systems predicted which clients would move from testing to full deployment, letting sales teams focus on the highest‑potential accounts.
That precision led to more predictable quarterly revenue, which in turn supported healthier stock growth. Investors cheered on these results, bidding up shares in anticipation of continued expansion. In C3.ai’s case, heavy R&D spending and the expenses of shifting to a usage‑based pricing model created a gap between top‑line gains and the bottom line.
Turning to a Consumption‑Based Revenue Model
Shifting from fixed subscriptions to a consumption‑based revenue model represented a bold experiment in AI‑infused business design. Charging by the hour of virtual CPU usage aligned C3.ai’s income more closely with real customer value — the more the software ran, the more revenue flowed in.
This approach tends to reduce churn, since clients keep using the platform only when they see clear benefits. Over time, however, average selling prices dipped and operating expenses rose, illustrating how any AI‑driven strategy can produce unexpected financial results when customer behavior changes or infrastructure costs spike.
Pros of AI Stock Prediction
Speed is one of the main advantages of AI in trading. Such systems can pore over financial filings, headlines, charts, and social media chatter in a matter of seconds. It would require a team of analysts working 24/7 to even come close.
Pattern recognition is another area where AI excels. It identifies slight correlations and long-term trends that human traders would probably never catch, especially over huge datasets that span years or even decades. These insights can surface opportunities early, often before the broader market catches on.
Another major advantage?AI doesn’t get emotional. It doesn’t panic when markets go down or get overconfident during a bull run. It stays with the data, eliminating the fear and greed that frequently derail human choices.
And AI models have the ability to backtest thousands of possible scenarios, continually sharpening their predictions. The system learns, adapts, and fine-tunes its method when new data arrives. This feedback loop keeps the model sharpened — unless, of course, it’s making the rounds of faulty data.
Cons and Limitations
Financial markets are chaotic, unpredictable, and often driven by emotion or unexpected events. When the script changes, AI systems can struggle.
Another problem is often over-fitting. That’s when a model picks up patterns that only existed in the past but fall apart in real time. If the system trains too narrowly on old data, it may miss the signals that matter most today.
There’s also the “garbage in, garbage out” issue. If a model’s training data is inaccurate, biased, or out of date, its predictions will often be off.
Another major concern is transparency. Some AI models are so sophisticated that even their creators don’t fully understand how they make decisions. That black box nature can make it difficult to trust AI when it comes to the output, especially when you are risking real money.
One other problem that is difficult to ignore is manipulation. AI systems that follow online sentiment or social trends can be deliberately misled through organized campaigns — bot activity, fake news, or viral hype can all throw things off.
Should You Trust AI Stock Predictions?
The short answer: sometimes. AI tends to work best with short-term price predictions and highly liquid stocks, especially when those stocks have lots of available data. In those cases, the models can deliver useful, probability-based insights.
But there are times when you should be cautious. AI struggles with black swan events — things like geopolitical surprises, pandemics, or sudden policy shifts that have no precedent. It also has a harder time with small-cap or thinly traded stocks, where the data pool is too shallow to build a reliable model.
At the end of the day, AI is a tool, not a guarantee. You can use it to filter ideas, confirm trends, and surface opportunities. But don’t turn off your brain. Combine AI insights with your own judgment, solid research, and a basic understanding of the business behind the stock.
While AI can help you move faster, you’re still the one making the call.
The Road Ahead for AI in Finance
Looking forward, expect tighter regulatory scrutiny of AI‑driven guidance as the SEC weighs in on algorithmic earnings forecasts. Generative models may soon automate financial reporting, writing earnings summaries and investor decks in minutes.
At the same time, the push for explainable AI will grow louder; you’ll want tools that reveal their assumptions, not lock you into opaque algorithms. Ethical protocols around AI‑driven trading communications will follow, ensuring predictive tools serve investors without misleading them.
Through it all, remember that AI stock prediction is not a magic bullet. It’s a lens that can bring hidden opportunities into focus, but only when you use it alongside solid risk management and your own market savvy.
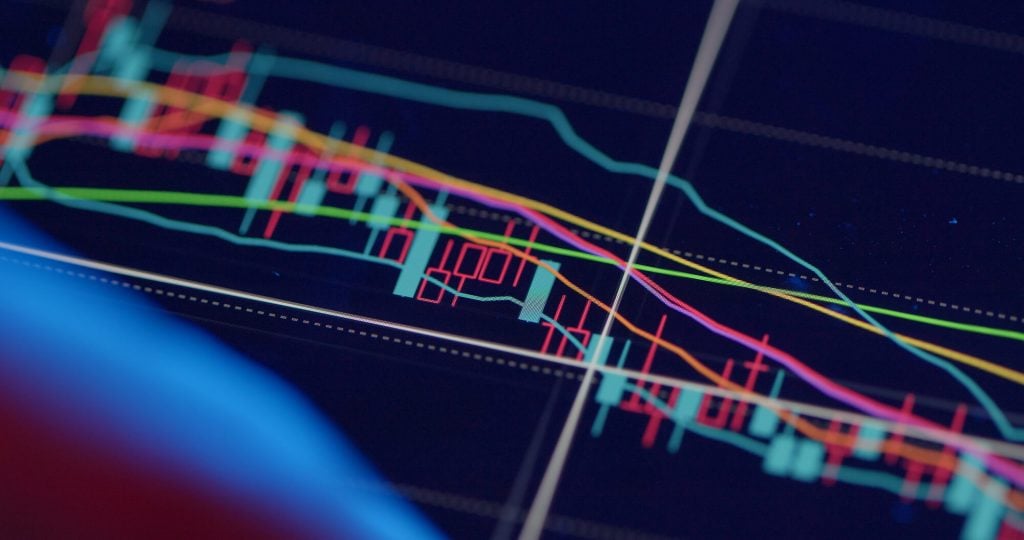
Become a Better Trader With Gorilla Trades
Gorilla Trades can help you spot high‑potential stocks and set smart stop‑loss levels. Whether you’re just starting out or you’ve been trading for years, we offer a 30‑day free trial so you can test how AI‑driven models and expert guidance blend into your strategy. Sign up today.